Everyone’s talking about RAG—it’s a huge deal in AI right now.
Consider a large retailer like Amazon, with a vast product catalog and a constantly evolving set of policies. A traditional AI-powered chatbot might struggle to provide up-to-date answers without manually retraining the model. Whereas, a retrieval augmented generation AI (RAG) powered chatbot can instantly retrieve product details, such as availability, reviews, pricing, and delivery options, from the company’s vast knowledge base and generate accurate, relevant responses. This approach ensures that the bot not only understands and processes the query but also provides precise, real-time information, improving customer satisfaction and reducing the need for human intervention.
RAG’s combination of retrieval and generative capabilities makes it ideal for situations where the latest information is crucial, ensuring answers are both current and contextually appropriate.
What is Retrieval Augmented Generation?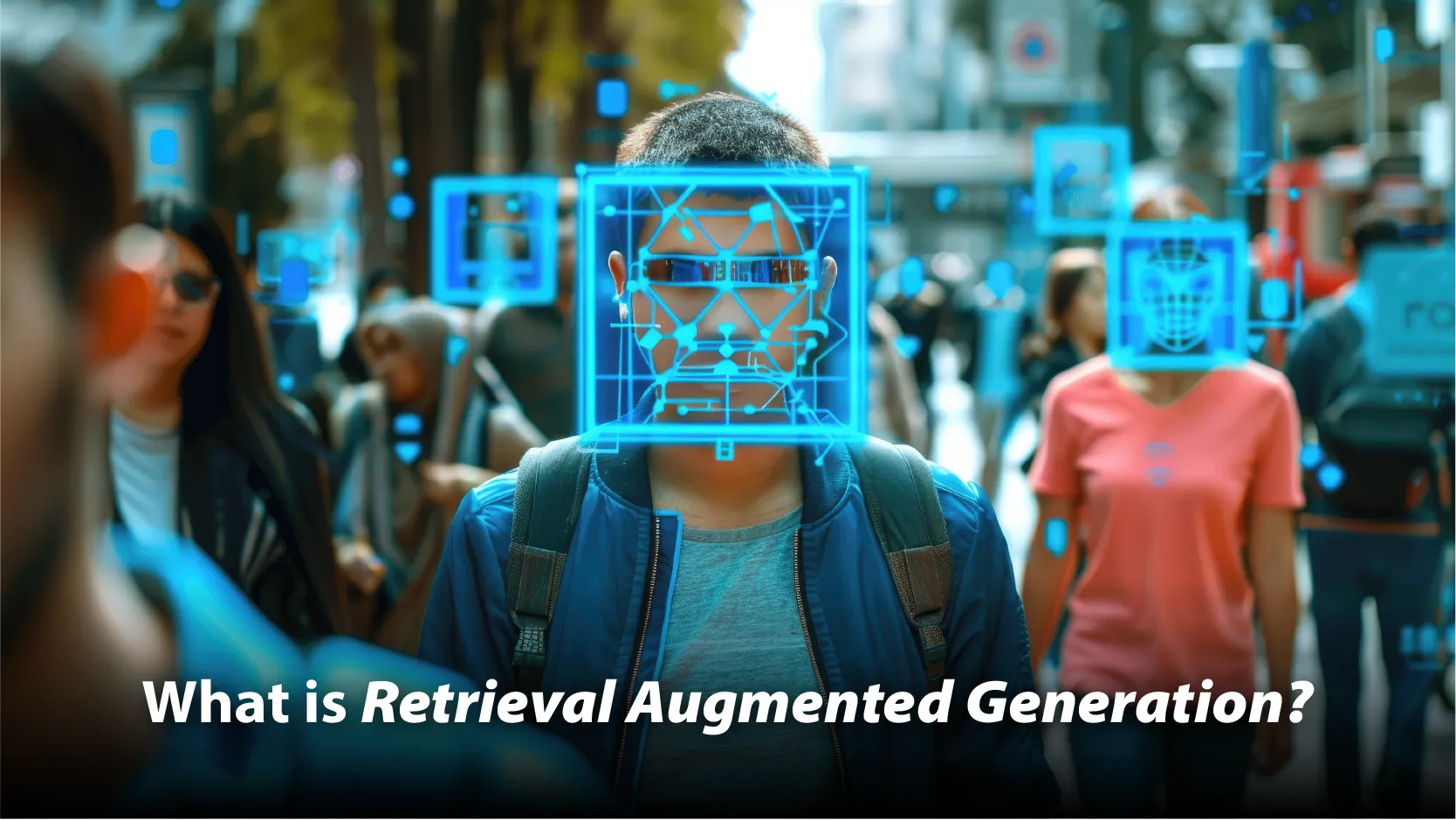
Retrieval augmented generation, or RAG for short, is an architectural approach that leverages bespoke data to boost the efficiency of large language model (LLM) applications. To do this, suitable documents and data are obtained and provided to the LLM as context for a task or inquiry. RAG has seen success with supporting chatbots and Q&A systems that need to retrieve domain-specific knowledge or remember up-to-date information.
Businesses who have used RAG have seen advancements like:
- A 35% rise in accuracy of responses
- a 25% increase in customer satisfaction
- a 50% decrease in the typical time needed to respond to consumer inquiries
Importance of Retrieval Augmented Generation
LLMs are a mainstay of artificial intelligence (AI) and are used in intelligent chatbots and other natural language processing (NLP) applications. The goal is to create bots that can answer questions from users in all kinds of situations by cross-referencing trustworthy knowledge sources. However, because of the nature of LLM technology, LLM responses become erratic. Additionally, LLM training data introduces a set cut-off date for the information it contains.
Known difficulties faced by LLMs include:
- Giving misleading information when the solution is not available.
- Giving the consumer generic or outdated information when they’re expecting a precise, up-to-date response.
- Constructing a reply using unreliable sources.
- When various training sources use the same vocabulary to discuss distinct topics, it might lead to erroneous responses owing to terminology confusion.
Consider the LLM as an overly enthusiastic new hire who refuses to keep up with current affairs but will always respond to inquiries with complete assurance. Unfortunately, this kind of behavior might undermine user confidence, so you don’t want your chatbots to adopt it!
RAG is one method for addressing some of these issues. By using pre-selected, reliable knowledge sources, it reroutes the LLM to obtain relevant information. Users receive insights into the LLM’s response generation process, and organizations have more control over the generated text output.
Also Read: 10 Ways Blockchain and AI Can Complement Each Other
Benefits of Retrieval Augmented Generation
There are several important advantages to the RAG approach, such as:
Delivering precise and accurate responses: RAG makes certain that an LLM’s reaction isn’t solely based on outdated, old training data. Rather, current external data sources are used by the model to obtain its answers.
Lowering false positives, or hallucinations: By supporting the output of the LLM model with significant, supplementary data, RAG seeks to lessen the likelihood of reacting with inaccurate or misleading information (also known as hallucinations). 87% of company executives believe RAG is a useful tool for enhancing LLMs and preventing hallucinations, according to a 451 Research poll.
Providing relevant, domain-specific responses: The LLM will be able to respond with contextually appropriate information that is customized to an organization’s private or domain-specific data by utilizing retrieval augmented generation AI.
Being effective and economical: When it comes to adding domain-specific data to personalize LLMs, RAG is less expensive and easier to use than other approaches. Organizations do not need to customize the model in order to implement RAG. This is especially useful when models need to have updated information fed to them often. An online retailer saw a 30% drop in running costs, which it attributed to improved query response times.
Applications of Retrieval Augmented Generation
RAG has a wide variety of usage scenarios. The most typical ones are:
● Chatbots for questions and answers:
LLMs can automatically extract more detailed responses from corporate documents and knowledge databases by combining them with chatbots. Chatbots are used to automate customer support and online lead follow-up so that issues may be resolved quickly and questions are answered.
● Search Augmentation:
By adding LLM-generated answers to search results, search engines may better answer informational queries and make it easier for users to get the information they need to complete their activities.
● Knowledge engine – question your data (such as HR records and compliance paperwork):
Employers may simply provide answers to their queries about benefits and regulations, security and compliance, and other HR-related topics by using company data as context for Learning Management Systems (LLMs).
Final Take on Retrieval Augmented Generation AI
RAG provides an efficient means of personalizing AI models, assisting in ensuring that outputs reflect current organizational knowledge, industry best practices, and online information. Retrieval augmented generation stands at the forefront of AI innovation. It opens the door to a new era of intelligent, enlightening, and trustworthy AI applications that can adjust to our constantly changing environment by bridging the gap between massive knowledge sources and generative models.