In a time when milliseconds can change market results, companies are moving quickly. They are moving from old cloud systems to new decentralized ones. These new systems can process data right at the source. Enter Edge AI. It combines artificial intelligence with edge computing. This lets data be analyzed locally. As a result, it avoids the delays of centralized servers. This fusion goes beyond simple innovation. It’s changing how businesses use data. Global leaders must understand Edge AI’s transformative potential. It is a strategic necessity.
The Dawn of a Decentralized Intelligence Era
Traditional cloud computing changed the game, but it now faces challenges. It can’t keep up with today’s heavy data needs. Sending a lot of information to distant data centers can lead to delays. It can also create bandwidth bottlenecks and raise security risks. Edge AI solves these issues by putting AI models right into devices. This includes sensors, cameras, industrial machines, and vehicles. It allows for quick decision-making without needing remote systems.
Consider a global logistics company managing fleets across continents. Edge AI helps onboard systems track traffic, weather, and engine data instantly. It can reroute trucks just milliseconds before a highway closes. Early adopters show real gains in operational efficiency and customer satisfaction. This agility is not just a theory. In healthcare, wearable devices with Edge AI can spot irregular heart rhythms. They alert patients and doctors before problems worsen. This proactive approach can save lives.
Redefining Real-Time Responsiveness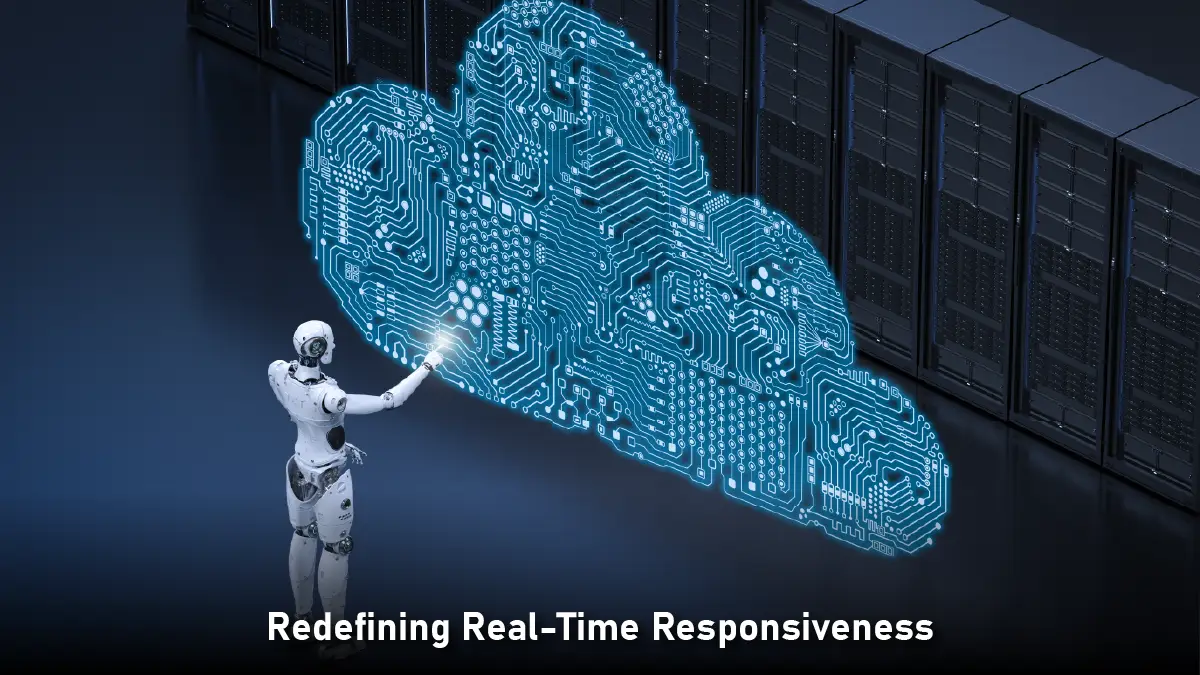
Edge AI shines in settings where speed and accuracy are essential. Take autonomous vehicles as an example. A self-driving car on city streets can’t wait for a cloud server. It needs to make quick decisions about braking or swerving right away. Edge AI processes data on-device. This cuts out network latency risks. So, it keeps working smoothly, even in areas with no connection.
Retail offers another compelling use case. Imagine smart shelves in a supermarket equipped with cameras and weight sensors. Edge AI algorithms keep an eye on inventory levels. They spot misplaced items and track customer interactions. Best of all, they do this without sending sensitive video feeds to the cloud. This makes restocking faster and keeps consumer privacy safe. Privacy is a growing worry in regulated markets.
Operational Efficiency and Cost Dynamics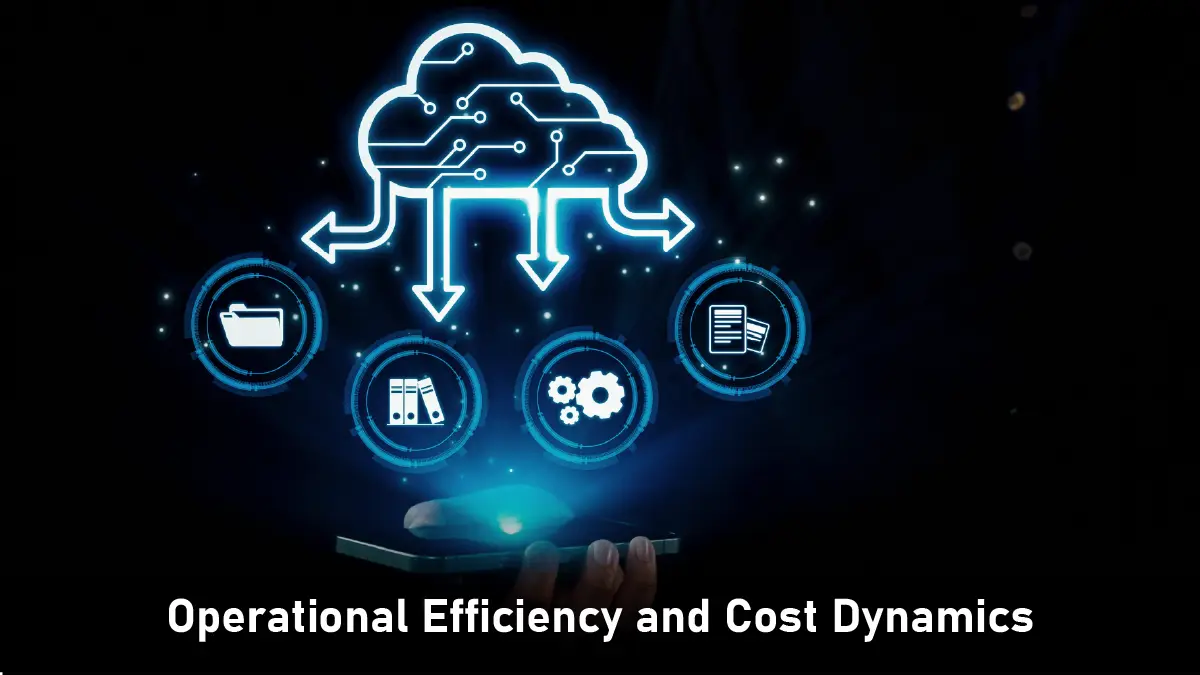
Edge AI’s value extends beyond speed. Enterprises cut costs by relying less on cloud infrastructure. This also lowers bandwidth and data transmission expenses. A manufacturer uses Edge AI for predictive maintenance. They check machinery vibrations and temperatures right onsite. Localized AI models flag anomalies and send maintenance alerts. This prevents unplanned downtime. So, instead of streaming terabytes of raw data to the cloud, you can save time and resources. Early adopters in heavy industries report fewer production stops. They also see longer equipment lifespans. This change saves millions each year.
According to industry data, predictive maintenance using AI can reduce maintenance costs by up to 25% and unexpected downtime by as much as 30% by the end of 2024.
Energy sectors are also capitalizing on this shift. Wind farms use Edge AI to improve turbine performance. It works by analyzing real-time weather data. Operators adjust blade angles and power output. This helps them maximize energy generation and reduce wear and tear. This local data processing is crucial in industries where profits rely on accuracy.
Also Read: Physical AI: Bridging the Gap Between Digital Intelligence and the Real World
Security and Compliance Reimagined
Data sovereignty and privacy rules, like GDPR and CCPA, make cloud workflows tricky. This is especially true for multinational companies. Edge AI solves these problems by handling sensitive data locally. This way, it meets regional data residency laws. A financial institution can use Edge AI in ATMs. This helps detect fraud while keeping customer data safe from outside networks. This local processing boosts security and builds consumer trust. This trust is valuable in fields like banking and healthcare.
Moreover, Edge AI reduces attack surfaces. Distributed edge networks protect data by splitting it up. This is different from centralized clouds, which can draw in cybercriminals. Even if one device is compromised, the breach remains isolated, preventing systemic failures. For industries handling intellectual property or sensitive information, this architectural resilience matters a lot.
Navigating Implementation Challenges
Despite its promise, Edge AI adoption isn’t without hurdles. Legacy infrastructure often doesn’t have the power for advanced AI models. So, hardware upgrades are needed. Also, managing decentralized systems needs strong orchestration tools. These tools help keep things consistent across many edge nodes.
Talent gaps further complicate deployments. Edge AI skills mix data science, IoT engineering, and cybersecurity. This blend is rare in today’s separated job market. Smart organizations are tackling this issue. They are partnering with schools or investing in training programs. Some use low-code AI platforms. This helps non-technical teams create simple models at the edge.
Interoperability remains another critical concern. Edge ecosystems include many devices and protocols. For smooth integration, we need open standards. These frameworks must work with any vendor. Industry groups are forming to address this fragmentation. They support unified architectures to make scalability easier.
The Road Ahead: Edge AI as a Competitive Catalyst
With better connectivity from 5G and satellite internet, Edge AI will become even stronger. Smart cities will use edge networks. These networks manage traffic, check pollution, and respond to emergencies right away. AI-driven drones will also analyze soil health and crop yields in farming. This will allow for precision farming on a larger scale than ever before.
For enterprises, the question isn’t whether to adopt Edge AI, but how fast. Early movers are changing the industry standards. Slower competitors struggle with old models. To stay ahead, leaders must:
Audit Infrastructure Readiness: Assess existing hardware and connectivity gaps.
Focus On High-Impact Use Cases. These are situations where latency or data sensitivity calls for edge deployment.
Build Strategic Partnerships: Team up with edge platform providers and AI innovators. This will help speed up timelines.
Invest in Talent Ecosystems: Create teams skilled in AI, IoT, and edge architectures.
Beyond the Hype
Edge AI isn’t a silver bullet; it’s a strategic enabler. Its true value lies in augmenting human decision-making, not replacing it. Businesses gain agility and innovation by combining local knowledge with human skills.
The transition demands vision, investment, and patience. For organizations ready to tackle the challenges, Edge AI promises a future. Here, data is processed quickly and turned into useful insights almost instantly. In this new paradigm, real-time isn’t an aspirational goal; it’s the baseline for survival.
As industries come together and competition grows, one thing is clear: the advantage goes beyond just technology. It’s the new battleground for global leadership. Companies that find this balance will lead their fields. Those that wait may become outdated. The time to act is now.