In the complex world of healthcare administration, medical coding is a key support. Imagine Maria, a skilled coder, bent over her desk at 2 a.m. She squints at a doctor’s quick, messy notes. Her task? Translate terms like ‘acute exacerbation of COPD’ into specific billing and insurance codes. This ensures compliance. Just one wrong digit can slow down patient care. It might also lead to audits or cost the hospital millions. Picture a tool that eases this burden. It can turn coding from a hurdle into a valuable asset. Enter Natural Language Processing (NLP). This technology is changing medical coding. It does so with speed, accuracy, and intelligence like never before.
The Hidden Cost of Manual Coding
Medical coding plays a vital role in healthcare. However, it faces significant challenges.
More than 20% of claim denials come from coding mistakes. This costs U.S. health systems about US$ 262 billion each year in wasted administration costs. Coders deal with a large volume of unstructured clinical notes. This makes their job tough. They must manually pull out diagnoses, procedures, and modifiers from long texts. It can feel like a never-ending task. The result? Delayed reimbursements, burnout, and a system perpetually playing catch-up. What if machines could read and understand clinical documents as well as, or even better than, humans? NLP is a part of artificial intelligence. It helps computers understand human language. NLP systems analyze context, meaning, and clinician intent. They turn unstructured text into useful data on a large scale. For healthcare leaders, this isn’t just small progress. It’s a huge change for operational resilience.
How NLP Decodes the Undecodable
At its core, NLP mimics human comprehension but with machine precision. Traditional rule-based coding software relies on keyword matching—ineffective when a physician documents ‘heart attack’ instead of ‘myocardial infarction.’ NLP goes deeper, using contextual analysis to infer meaning. For example, consider this sentence from a patient chart: “The patient presented with chest pain radiating to the left arm, troponin levels elevated; STEMI confirmed.”
An NLP algorithm parses this like a seasoned coder:
- Identifies ‘STEMI’ (ST-elevation myocardial infarction) as the primary diagnosis.
- Links ‘chest pain’ and ‘elevated troponin’ as supporting evidence.
- Maps findings to ICD-10 code I21.3 (ST-elevation myocardial infarction of unspecified site).
This ability to ‘read between the lines’ is why institutions like Mayo Clinic report a 40% reduction in coding backlogs after implementing NLP-driven tools.
The Tangible Impact: Efficiency, Accuracy, and Beyond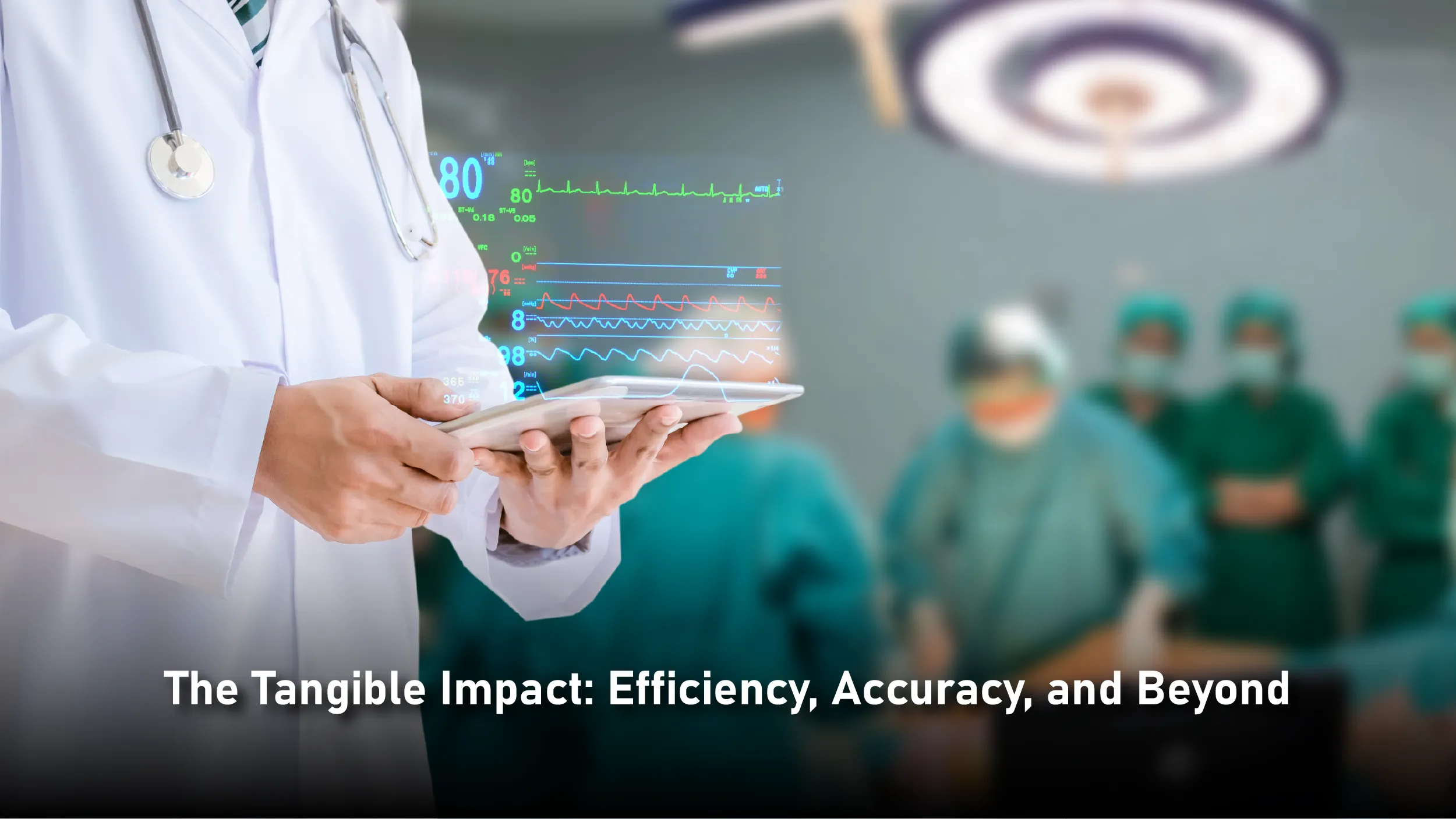
Slashing Administrative Bloat
At Johns Hopkins Hospital, NLP reduced coding turnaround time from 14 days to 48 hours. By automating routine cases, coders now focus on complex charts, driving a 25% productivity boost. “It’s like having a co-pilot who never sleeps,” says Dr. Linda Carter, Chief Medical Officer. “Our teams are reclaiming hours once lost to grunt work.”
Curbing Costly Errors
Human coders have a 95% accuracy rate. That sounds good, but a 5% error rate leads to US$ 13 billion in annual denials. In contrast, NLP systems, trained on millions of coded records, reach almost perfect accuracy. At Houston Methodist, NLP identified inconsistencies in US$ 122.8 million of potential claim rejections.
Closing the Revenue Cycle Gap
A 2023 study in Health Affairs revealed that hospitals using NLP accelerated revenue cycles by 18 days on average. Faster coding means faster claims submission, fewer denials, and healthier cash flow—a lifeline for margins squeezed by inflation and staffing shortages.
Future-Proofing Compliance
With ICD-11 looming, coding guidelines will grow more complex. NLP systems adapt in real time, updating code mappings and flagging documentation gaps. During a recent CMS audit, NYU Langone credited NLP with identifying 300+ documentation discrepancies before they escalated to penalties.
Also Read: 8 Ways Patient Engagement Technology Improved Patient Care
Case Study: How NLP Rescued a Rural Health System
Take St. Luke’s Regional Health, a 150-bed hospital in Iowa. Plagued by coder shortages and a 22% denial rate, they partnered with an NLP vendor to overhaul their workflow. Within six months:
- Coding errors dropped by 62%.
- Denials decreased to 9%, reclaiming $1.2 million annually.
- Coders’ overtime hours vanished, reducing turnover from 40% to 12%.
“NLP didn’t replace our team—it empowered them,” says CFO Mark Thompson. “We’re now coding 100% of charts within 24 hours, something we thought was impossible.”
Navigating the Roadblocks: What Leaders Must Consider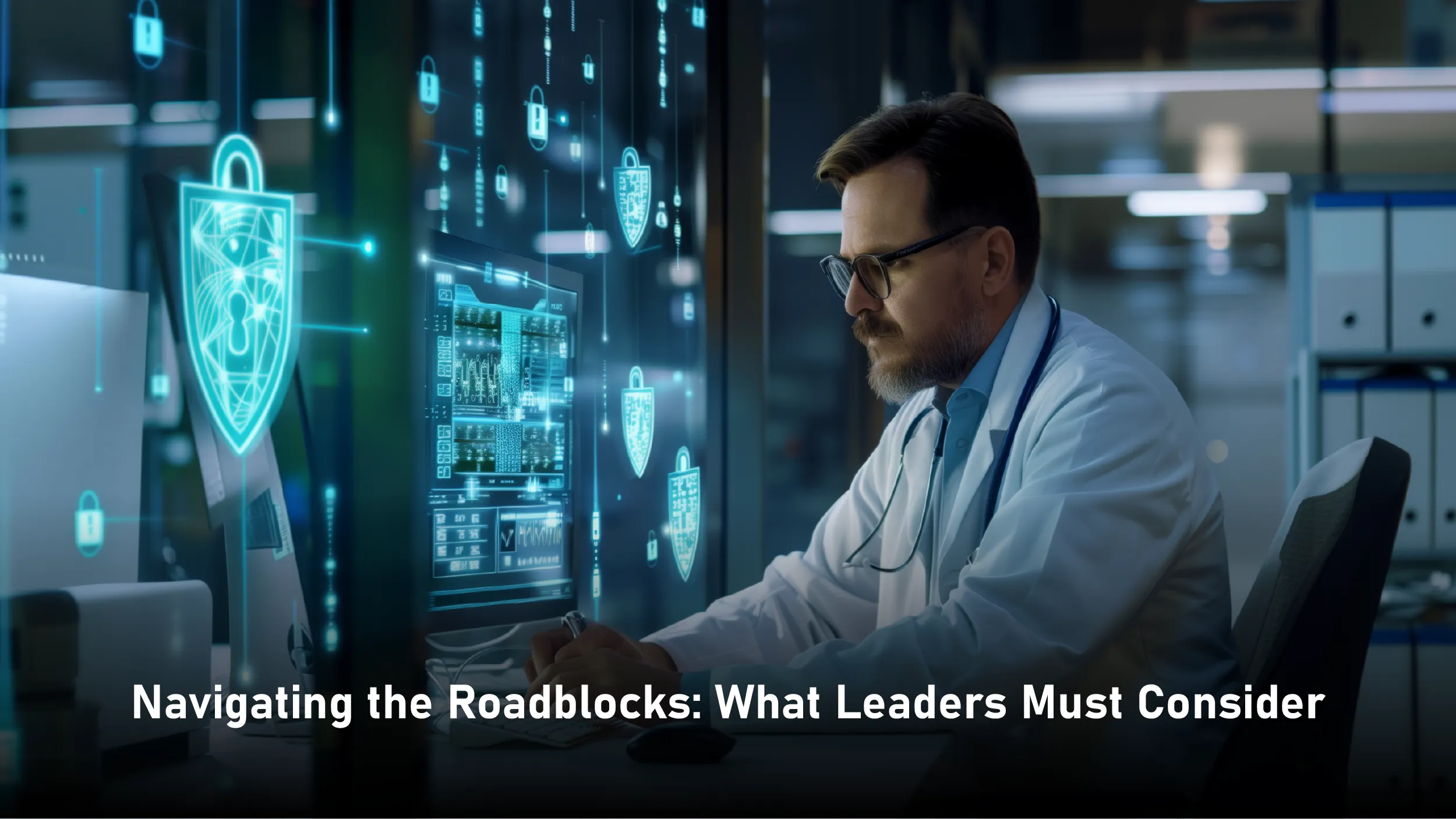
While NLP’s promise is undeniable, its adoption isn’t without hurdles.
Data Privacy and Security
NLP systems ingest vast amounts of PHI (Protected Health Information). Leaders must ensure vendors comply with HIPAA and HITRUST standards, encrypting data both at rest and in transit.
Integration with Legacy Systems
Many EHRs weren’t built with AI in mind. API-driven NLP platforms, like those used by Kaiser Permanente, offer plug-and-play compatibility, minimizing IT bottlenecks.
Clinician Buy-In
Some physicians fear NLP could misinterpret nuances. Proactive training—and transparent feedback loops—alleviate concerns. At Cleveland Clinic, coders review NLP outputs alongside clinicians, fostering trust.
Regulatory Uncertainty
The FDA’s evolving stance on AI in healthcare warrants caution. Partnering with vendors who prioritize regulatory readiness, like Epic or 3M, mitigates risk.
The Future of Coding
Tomorrow’s NLP tools won’t just code—they’ll predict. Imagine an algorithm analyzing a surgeon’s note to flag undercoded procedures (“Did you miss the bilateral modifier?”) or suggesting documentation tips to maximize reimbursement. Startups like CodaMetrix are already piloting such systems, blending NLP with predictive analytics to turn coders into strategic advisors.
A Blueprint for Implementation
Assess Workflow Gaps
Audit where coding delays or errors hurt most. Is it emergency department charts? Surgical coding? Prioritize high-impact areas.
Pilot Before Scaling
Run a 90-day pilot with one department. Measure metrics like time-per-chart, denial rates, and coder satisfaction.
Choose the Right Partner
Opt for vendors with healthcare-specific NLP expertise, like Nuance or Optum. Demand transparency in model training data and accuracy benchmarks.
Upskill Your Team
Transition coders from data entry to data governance. Certifications in AI oversight or NLP auditing future-proof their roles.
Monitor and Iterate
Regularly review NLP outputs for biases or inaccuracies. Update models with new code sets and clinical terminology.
Conclusion
The rise of NLP in medical coding isn’t about replacing humans—it’s about amplifying their potential. For healthcare leaders, this technology offers a rare trifecta: financial stability, operational agility, and enhanced compliance. But beyond spreadsheets and ROI, it’s a step toward a system where clinicians spend less time documenting and more time healing.
As Maria, our veteran coder, now reflects: “NLP handles the monotony. I handle the mysteries. Together, we’re coding a future where healthcare runs as it should—smoothly, smartly, and sustainably.”
For executives ready to lead this charge, the message is clear: The future of medical coding isn’t in a coder’s manual. It’s in the language we speak, the data we generate, and the machines that help us make sense of it all.