As AI advances quickly, worries about its centralization are intensifying. Decentralized AI (DAI), in response, is becoming increasingly popular.
Historically, centralized tech giants like Google and OpenAI have dominated AI research and use. These centralized systems often need large data and funds. This raises concerns about data privacy, transparency, bias, and power concentration.
A novel strategy, called DAI, is gaining traction. It aims to transform AI by distributing governance, model training, and data processing across a network of nodes. This would avoid concentrating power in one place. So, what is it and why is it important? Let’s find out.
What Is Decentralized AI?
Decentralized AI processes, stores, and manages data across multiple nodes instead of depending on a single authority by using distributed networks and blockchain technology. This method enables individual users to gain from AI without giving up control or privacy over their data. Decentralized AI systems process data on local devices. It’s done safely and independently, without sending it to a central server.
Decentralized AI models create a transparent, trustless environment for AI. They use technologies like federated learning, blockchain, smart contracts, homomorphic encryption, and dApps.
What are the key features of decentralized AI?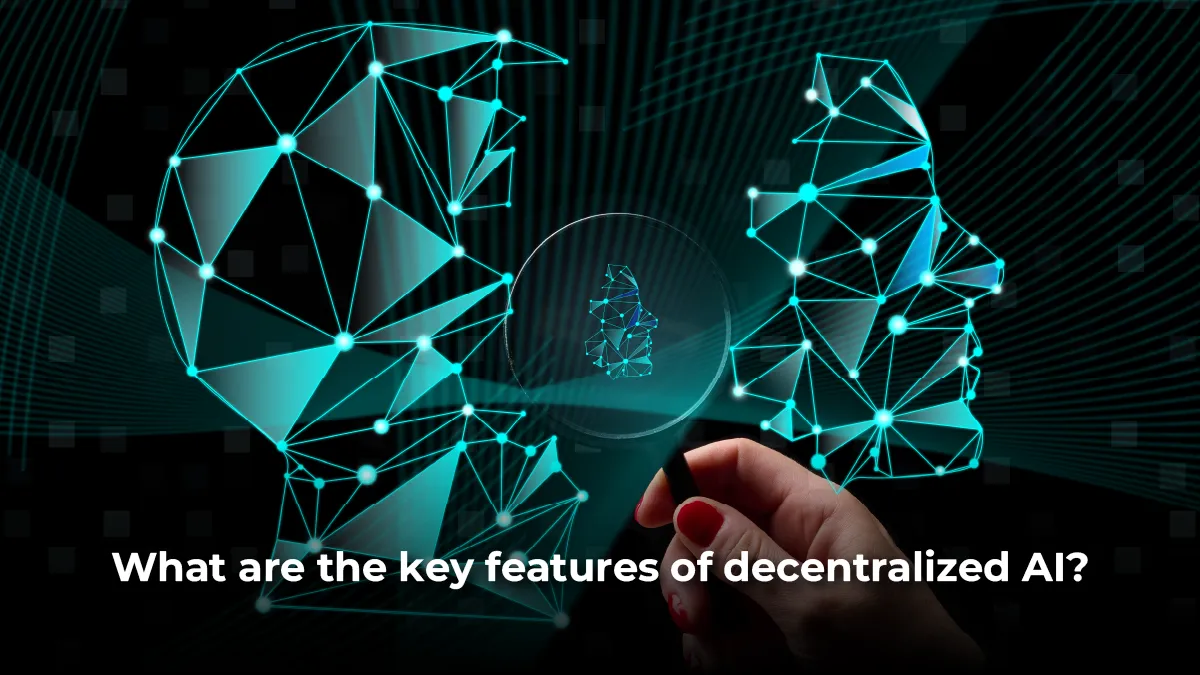
- Distributed ledgers and blockchain: Blockchain technology supports DAI. It provides an unchangeable, transparent ledger to monitor AI operations and data transfers. Accountability, security, and tamper-proof activities are guaranteed by this decentralized ledger.
- Federated learning: It trains AI models on decentralized devices. This protects the privacy of the data. In order to improve global models and preserve a decentralized structure, model updates are communicated back to the main network.
- Smart contracts: They automate agreements in decentralized AI systems. These self-executing contracts boost cooperation and eliminate middlemen.
- Homomorphic encryption: It protects user privacy and guards against unauthorized access. It allows the processing of data while it is still encrypted.
- Decentralized apps: dApps, which frequently integrate open-source development and collaboration. They let people build and run decentralized AI solutions without central control.
Also Read: What Data Analytics Automation Means for Your Business Growth
Benefits of Decentralized AI
● Improved Privacy and Security
Better security and privacy are two of the main advantages of DAI. Traditional AI systems raise privacy concerns. They often ask users to share data with a central authority. Conversely, decentralized artificial intelligence gives people more control over their data. DAI cuts the risk of data breaches by processing data locally. It only shares the required insights.
● Enhanced Transparency and Credibility
Decentralized AI encourages transparency by logging all transactions and decisions on a blockchain. This immutable ledger improves accountability. It ensures that every AI action can be tracked and validated. Because users can see how their data is used and decisions are made, this kind of transparency is essential to gaining their trust.
● Democratized Development of Artificial Intelligence
DAI lets smaller organizations and independent researchers join AI development. Abandoning centralized control allows more contributors to join. This encourages diversity and innovation in AI research. A wider range of demands and use cases may be addressed by more inclusive and diverse AI models as a result of this democratization.
● Fault Tolerance and Resilience
Data and tasks are distributed among several nodes in a decentralized AI network. Its architecture makes the system more resistant to attacks and bugs. It lowers the risk of a single point of failure. The AI system is stable and dependable. Other nodes can work even if one fails or is compromised.
● Encouraged Involvement
Incentives are frequently used by decentralized AI networks to promote participation. Several platforms give crypto tokens to users. They are based on the value users add to the network. This encourages high-quality contributions and creates a competitive environment that propels ongoing advancements in AI capabilities.
● Training AI models on a Decentralized network
Decentralized AI training leverages blockchain to distribute AI training across multiple nodes, ensuring data security and integrity without relying on centralized repositories. Each node trains the AI model on distinct data subsets, enhancing privacy. Depending on the protocol, updated model parameters are either pooled in a decentralized manner or sent to a central node, with blockchain ensuring secure aggregation and fair reward for node contributions.
Challenges with Centralized AI Models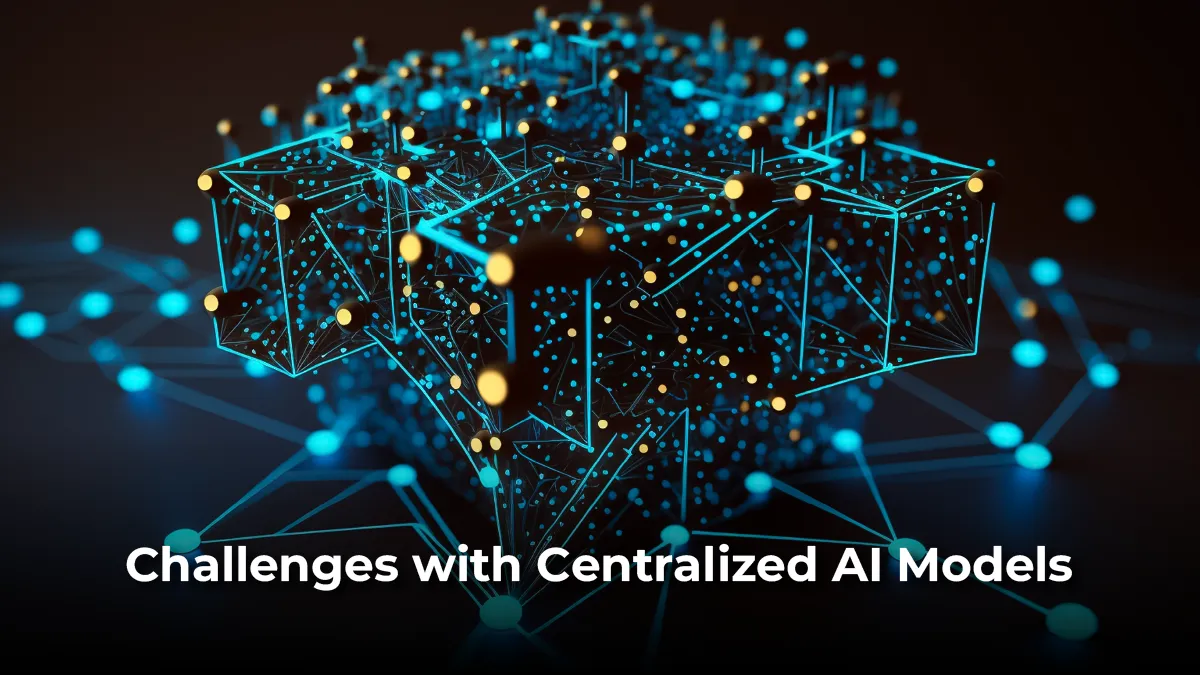
The problems with centralized AI models are as follows:
- With their vast data sets and expensive data science teams, giant companies create a cycle in which created models produce large amounts of data. This enhances their datasets and solidifies their leadership in the creation of AI models.
- Current AI systems often rely on a single source. This leads to biased and insufficient knowledge.
- Transparency about the amount of data consumed is lacking in centralized AI systems. Large corporations use user data without being required to disclose it.
Popular DAI Projects
Although DAI is still in its infancy, interest is already increasing. There are a number of upcoming initiatives that make use of DAI and its potential. Here are a few notable instances:
1. OpenMined
A non-profit group called OpenMined is committed to helping AI researchers understand and predict how AI will affect society. It has created AI governance infrastructure using technologies that improve privacy. It gives scholars access to datasets and AI systems for in-depth research on the worldwide effects of AI.
OpenMined has a unique approach. It combines blockchain technology with cryptographic methods, like multi-party computation and homomorphic encryption. Consequently, machine learning models can be trained using private user data.
2. Ritual
Ritual is a platform for decentralized AI computing. It aims to create an incentive-based network that links distributed computing devices.
Its five main focuses are:
- Powering hosting, sharing, and fine-tuning of AI models.
- Providing an API for model access.
- Ensuring computational integrity and censorship resistance.
- Protecting privacy.
- Supporting inference.
Ritual’s platform offers an API layer for user access and facilitates the fine-tuning of AI models. Ritual introduces a novel architecture with a crowdsourced governance layer. It addresses safety, finance, alignment, and model progression. Overall, it is the network for open AI infrastructure.
Bottom Line
Decentralized AI promises a better future for AI. It offers improved security, transparency, and democratization of AI development. It also faces tough obstacles, like regulatory barriers, scaling issues, and complexity. Decentralized models could revolutionize the AI space. It may make it more inclusive and resilient as technology advances and new apps emerge.